Prepara a tabela de dados
# glimpse(teca_arv)
# glimpse(teca_qui)
# glimpse(teca_crapar)
# glimpse(teca_gran)
# Junções.
tb <- full_join(teca_qui, teca_crapar)
## Joining, by = c("loc", "cam")
tb <- inner_join(tb, teca_arv[, c("loc", "prod")])
## Joining, by = "loc"
tb <- as_tibble(tb)
glimpse(tb)
## Observations: 150
## Variables: 24
## $ loc <int> 1, 1, 1, 2, 2, 2, 3, 3, 3, 4, 4, 4, 5, 5, 5, 6, 6, 6…
## $ cam <fct> "[0, 5)", "[5, 40)", "[40, 80)", "[0, 5)", "[5, 40)"…
## $ ph <dbl> 6.8, 6.7, 6.7, 4.7, 4.7, 4.9, 7.6, 6.8, 6.9, 6.6, 6.…
## $ p <dbl> 22.51, 0.83, 0.01, 3.89, 0.69, 0.10, 11.52, 1.66, 0.…
## $ k <dbl> 72.24, 13.42, 7.23, 48.13, 12.34, 8.64, 114.55, 25.8…
## $ ca <dbl> 8.27, 2.91, 2.33, 0.97, 0.76, 0.21, 7.63, 3.22, 2.22…
## $ mg <dbl> 1.70, 1.77, 0.51, 0.16, 0.14, 0.00, 1.53, 1.31, 1.09…
## $ al <dbl> 0.0, 0.0, 0.0, 0.3, 0.6, 0.6, 0.0, 0.0, 0.0, 0.0, 0.…
## $ ctc <dbl> 12.47, 6.57, 4.52, 5.30, 4.17, 3.47, 12.41, 6.26, 5.…
## $ sat <dbl> 81.41, 71.74, 63.23, 23.66, 22.35, 6.69, 76.14, 73.4…
## $ mo <dbl> 72.2, 25.6, 9.7, 34.4, 8.7, 9.7, 50.4, 15.6, 9.5, 50…
## $ arg <dbl> 183.7, 215.0, 285.5, 231.9, 212.6, 237.0, 192.6, 234…
## $ are <dbl> 769.5, 749.5, 674.5, 741.0, 775.0, 752.0, 715.5, 698…
## $ cas <dbl> 1.8, 2.2, 3.7, 0.4, 1.1, 1.7, 8.4, 19.0, 14.3, 5.5, …
## $ acc <dbl> 769.93, 750.04, 675.69, 741.11, 775.25, 752.42, 717.…
## $ Ur <dbl> 0.22288275, 0.17845472, 0.18791987, 0.13107692, 0.16…
## $ Us <dbl> 0.6477212, 0.5799842, 0.6472682, 0.6967997, 0.572831…
## $ alp <dbl> -0.7242483, -0.8327602, -0.7221707, -0.5484938, -0.5…
## $ n <dbl> 3.589612, 3.112403, 3.250733, 4.006821, 2.917137, 2.…
## $ I <dbl> 0.8152153, 0.9572842, 0.8352604, 0.6201503, 0.690926…
## $ Ui <dbl> 0.4497358, 0.3956184, 0.4353160, 0.4306964, 0.387045…
## $ S <dbl> -0.34126593, -0.27326860, -0.32901803, -0.51467615, …
## $ cad <dbl> 0.2268530, 0.2171637, 0.2473961, 0.2996195, 0.222109…
## $ prod <dbl> 68.22631, 68.22631, 68.22631, 24.27449, 24.27449, 24…
# Trata valor alto de P.
tb$p[tb$p > 80] <- NA
# Empilha os valores das variáveis de solo.
tb_long <- tb %>%
gather(key = "variable", value = "valor", ph:cad)
# Protótipo.
gg <- ggplot(data = tb_long,
mapping = aes(x = valor, y = prod, color = cam)) +
facet_wrap(facets = ~variable, scales = "free_x", ncol = 3) +
geom_point(shape = 1) +
labs(color = "Camada") +
xlab("Valor da variável de solo") +
ylab("Produção de teca")
# Regressão linear simples.
gg +
geom_smooth(method = "lm",
formula = y ~ poly(x, degree = 2),
se = FALSE)
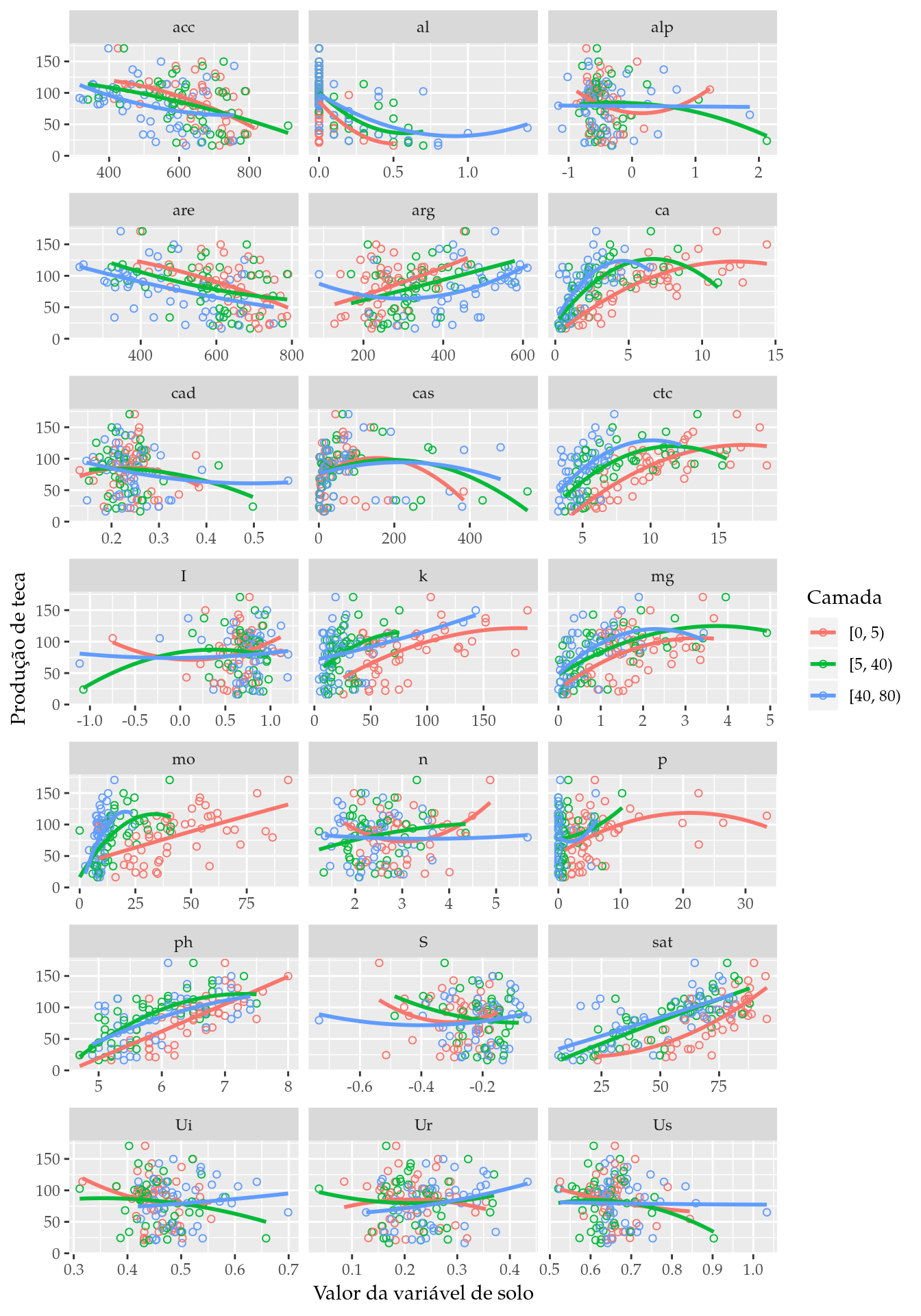
# Regressão quantílica.
gg +
geom_quantile(quantiles = 0.9,
formula = y ~ poly(x, degree = 2))
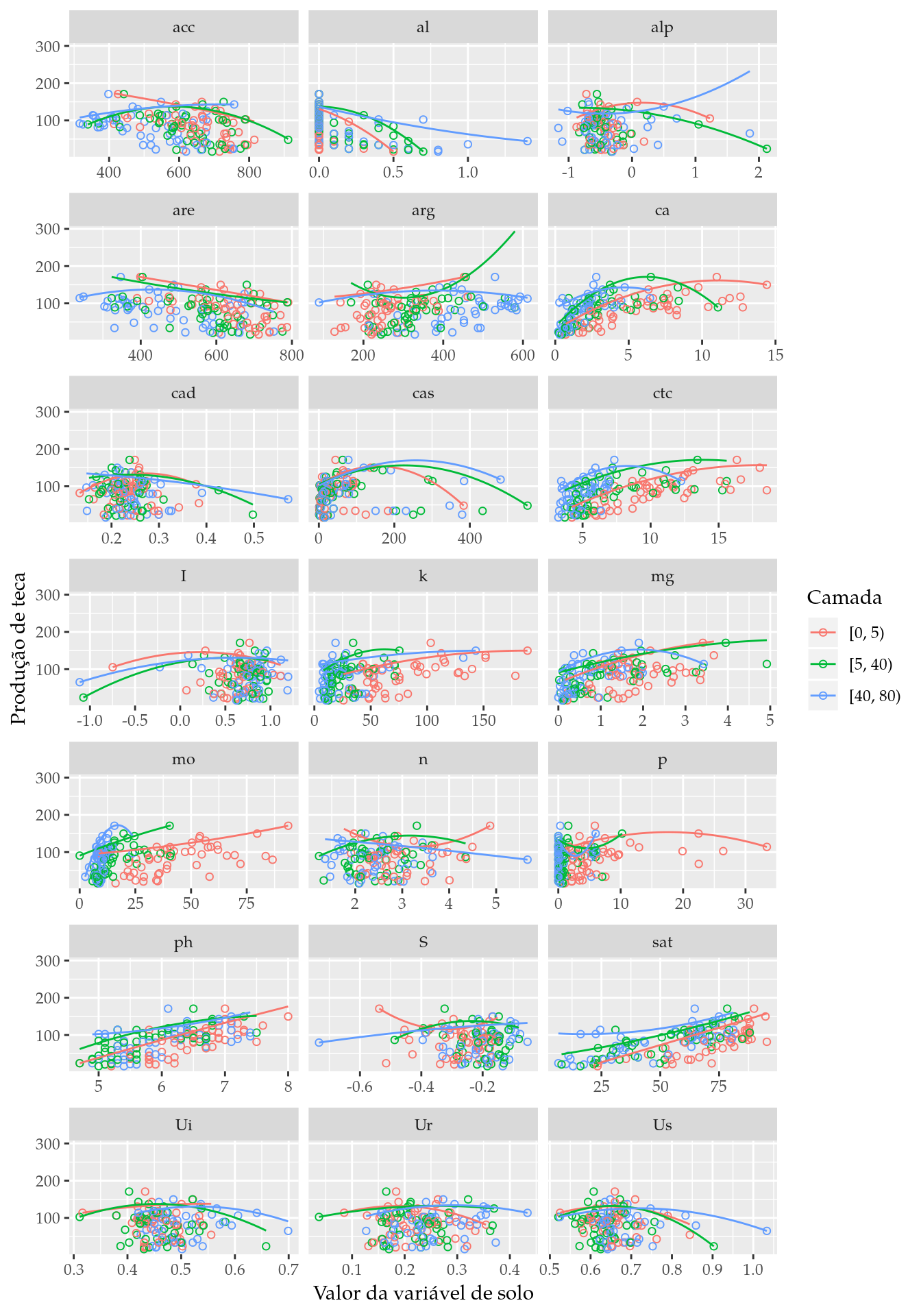
Regressão quantílica
tb_fit <- tb_long %>%
group_by(variable, cam) %>%
drop_na() %>%
nest()
# ATTENTION: o modelo 0 é reajustado em função dos missings que mudam de
# quantidade como função do split.
tb_fit <- tb_fit %>%
mutate(qtl_0 = map(data,
rq,
formula = prod ~ 1,
tau = 0.9),
qtl_1 = map(data,
rq,
formula = prod ~ valor,
tau = 0.9),
qtl_2 = map(data,
rq,
formula = prod ~ valor + I(valor^2),
tau = 0.9))
# # Quadro de anova para modelos encaixados.
# tb_fit <- tb_fit %>%
# mutate(anova_01 = map(qtl_1, anova, qtl_0),
# anova_12 = map2(qtl_2, qtl_1, anova))
extract_coef <- function(fit_work, fit_ref) {
if (missing(fit_ref)) {
p <- NULL
} else {
p <- anova(fit_work, fit_ref)$table$pvalue
}
b <- coef(fit_work)
c(b, pvalue = p) %>%
as.list() %>%
data.frame(check.names = FALSE)
}
# # Teste.
# extract_coef(tb_fit$qtl_2[[1]], tb_fit$qtl_1[[1]])
# extract_coef(tb_fit$qtl_1[[1]], qtl_0)
# Extrai as estimativas e valor p para criar tabela.
tb_result <- tb_fit %>%
transmute(variable = variable,
cam = cam,
null = map(qtl_0, extract_coef),
first = map2(qtl_1, qtl_0, extract_coef),
secon = map2(qtl_2, qtl_1, extract_coef),
) %>%
gather("model", "coef", -variable, -cam) %>%
unnest(coef)
tb_result
## # A tibble: 189 x 7
## variable cam model `(Intercept)` valor pvalue `I(valor^2)`
## <chr> <fct> <chr> <dbl> <dbl> <dbl> <dbl>
## 1 ph [0, 5) null 125. NA NA NA
## 2 ph [5, 40) null 125. NA NA NA
## 3 ph [40, 80) null 125. NA NA NA
## 4 p [0, 5) null 131. NA NA NA
## 5 p [5, 40) null 125. NA NA NA
## 6 p [40, 80) null 125. NA NA NA
## 7 k [0, 5) null 125. NA NA NA
## 8 k [5, 40) null 125. NA NA NA
## 9 k [40, 80) null 125. NA NA NA
## 10 ca [0, 5) null 125. NA NA NA
## # … with 179 more rows
# Organiza para melhor disposição da informação.
tb_result <- tb_result %>%
rename(beta_0 = "(Intercept)",
beta_1 = "valor",
beta_2 = "I(valor^2)") %>%
select(-pvalue, everything()) %>%
mutate(model = factor(model, levels = unique(model))) %>%
arrange(variable, cam, model)
tb_result
## # A tibble: 189 x 7
## variable cam model beta_0 beta_1 beta_2 pvalue
## <chr> <fct> <fct> <dbl> <dbl> <dbl> <dbl>
## 1 acc [0, 5) null 125. NA NA NA
## 2 acc [0, 5) first 243. -0.169 NA 0.0870
## 3 acc [0, 5) secon 222. -0.0836 -0.0000844 0.906
## 4 acc [5, 40) null 125. NA NA NA
## 5 acc [5, 40) first 156. -0.0437 NA 0.654
## 6 acc [5, 40) secon -137. 0.940 -0.000806 0.474
## 7 acc [40, 80) null 125. NA NA NA
## 8 acc [40, 80) first 88.7 0.0715 NA 0.391
## 9 acc [40, 80) secon 31.6 0.309 -0.000213 0.646
## 10 al [0, 5) null 125. NA NA NA
## # … with 179 more rows
# Ajustes significativos a 5%. Pega o maior modelo.
tb_signif <- tb_result %>%
filter(pvalue < 0.05) %>%
group_by(variable, cam) %>%
slice(n()) %>%
ungroup()
knitr::kable(tb_result)
acc |
[0, 5) |
null |
125.3399596 |
NA |
NA |
NA |
acc |
[0, 5) |
first |
242.7814910 |
-0.1692722 |
NA |
0.0869799 |
acc |
[0, 5) |
secon |
221.5909382 |
-0.0835741 |
-0.0000844 |
0.9057883 |
acc |
[5, 40) |
null |
125.3399596 |
NA |
NA |
NA |
acc |
[5, 40) |
first |
156.0849914 |
-0.0436577 |
NA |
0.6544456 |
acc |
[5, 40) |
secon |
-136.6867935 |
0.9395528 |
-0.0008058 |
0.4744945 |
acc |
[40, 80) |
null |
125.3399596 |
NA |
NA |
NA |
acc |
[40, 80) |
first |
88.6954207 |
0.0715023 |
NA |
0.3910965 |
acc |
[40, 80) |
secon |
31.5983069 |
0.3085285 |
-0.0002134 |
0.6455436 |
al |
[0, 5) |
null |
125.3399596 |
NA |
NA |
NA |
al |
[0, 5) |
first |
130.7281877 |
-170.7276572 |
NA |
0.3814584 |
al |
[0, 5) |
secon |
130.7281877 |
-131.9440885 |
-193.9178436 |
1.0000000 |
al |
[5, 40) |
null |
125.3399596 |
NA |
NA |
NA |
al |
[5, 40) |
first |
137.1213808 |
-105.5689730 |
NA |
0.0018894 |
al |
[5, 40) |
secon |
137.1213808 |
-5.6605508 |
-238.5353322 |
0.2474453 |
al |
[40, 80) |
null |
125.3399596 |
NA |
NA |
NA |
al |
[40, 80) |
first |
137.1213808 |
-66.2268338 |
NA |
0.0292740 |
al |
[40, 80) |
secon |
137.1213808 |
-94.0916949 |
19.9034722 |
0.5553745 |
alp |
[0, 5) |
null |
130.7281877 |
NA |
NA |
NA |
alp |
[0, 5) |
first |
147.2570076 |
30.9784959 |
NA |
0.0595835 |
alp |
[0, 5) |
secon |
146.5564189 |
12.1585501 |
-37.3017227 |
0.8361981 |
alp |
[5, 40) |
null |
130.7281877 |
NA |
NA |
NA |
alp |
[5, 40) |
first |
120.3809779 |
-28.6306365 |
NA |
0.0015285 |
alp |
[5, 40) |
secon |
125.2197392 |
-21.1110934 |
-12.5222173 |
0.5404658 |
alp |
[40, 80) |
null |
125.3399596 |
NA |
NA |
NA |
alp |
[40, 80) |
first |
131.2219618 |
5.7890335 |
NA |
0.6608027 |
alp |
[40, 80) |
secon |
122.2283874 |
18.9924755 |
21.7062767 |
0.6458951 |
are |
[0, 5) |
null |
125.3399596 |
NA |
NA |
NA |
are |
[0, 5) |
first |
239.9696595 |
-0.1734461 |
NA |
0.0510935 |
are |
[0, 5) |
secon |
239.2790786 |
-0.1704822 |
-0.0000031 |
0.9936331 |
are |
[5, 40) |
null |
125.3399596 |
NA |
NA |
NA |
are |
[5, 40) |
first |
206.0493722 |
-0.1312763 |
NA |
0.1886225 |
are |
[5, 40) |
secon |
242.8573946 |
-0.2544612 |
0.0000971 |
0.9132254 |
are |
[40, 80) |
null |
125.3399596 |
NA |
NA |
NA |
are |
[40, 80) |
first |
141.1759500 |
-0.0234781 |
NA |
0.7269071 |
are |
[40, 80) |
secon |
30.1449448 |
0.4977881 |
-0.0005789 |
0.2911675 |
arg |
[0, 5) |
null |
125.3399596 |
NA |
NA |
NA |
arg |
[0, 5) |
first |
84.2943767 |
0.1912636 |
NA |
0.1872307 |
arg |
[0, 5) |
secon |
108.5165147 |
0.0499888 |
0.0001940 |
0.7759759 |
arg |
[5, 40) |
null |
125.3399596 |
NA |
NA |
NA |
arg |
[5, 40) |
first |
79.5199105 |
0.1542883 |
NA |
0.2610368 |
arg |
[5, 40) |
secon |
322.2366116 |
-1.3774678 |
0.0022913 |
0.1331249 |
arg |
[40, 80) |
null |
125.3399596 |
NA |
NA |
NA |
arg |
[40, 80) |
first |
119.2573446 |
0.0269142 |
NA |
0.5051287 |
arg |
[40, 80) |
secon |
80.1683848 |
0.2836520 |
-0.0003707 |
0.4020180 |
ca |
[0, 5) |
null |
125.3399596 |
NA |
NA |
NA |
ca |
[0, 5) |
first |
58.6995879 |
8.7339937 |
NA |
0.0000799 |
ca |
[0, 5) |
secon |
28.2771531 |
24.0039433 |
-1.0808063 |
0.0338630 |
ca |
[5, 40) |
null |
125.3399596 |
NA |
NA |
NA |
ca |
[5, 40) |
first |
80.8817553 |
13.8068958 |
NA |
0.0000000 |
ca |
[5, 40) |
secon |
36.8470351 |
43.1311267 |
-3.4654478 |
0.0219065 |
ca |
[40, 80) |
null |
125.3399596 |
NA |
NA |
NA |
ca |
[40, 80) |
first |
99.0822352 |
11.8133993 |
NA |
0.1967138 |
ca |
[40, 80) |
secon |
86.0424446 |
24.0838810 |
-2.5530613 |
0.7871121 |
cad |
[0, 5) |
null |
130.7281877 |
NA |
NA |
NA |
cad |
[0, 5) |
first |
16.1061292 |
493.4425225 |
NA |
0.1607618 |
cad |
[0, 5) |
secon |
-64.6981709 |
1454.5346898 |
-2657.1934029 |
0.7374688 |
cad |
[5, 40) |
null |
130.7281877 |
NA |
NA |
NA |
cad |
[5, 40) |
first |
192.8750229 |
-243.3021733 |
NA |
0.4836707 |
cad |
[5, 40) |
secon |
63.1877896 |
571.4228015 |
-1198.2065064 |
0.6985334 |
cad |
[40, 80) |
null |
125.3399596 |
NA |
NA |
NA |
cad |
[40, 80) |
first |
162.2219522 |
-169.7106073 |
NA |
0.4153979 |
cad |
[40, 80) |
secon |
143.6439165 |
-37.1307391 |
-174.9621651 |
0.9907049 |
cas |
[0, 5) |
null |
125.3399596 |
NA |
NA |
NA |
cas |
[0, 5) |
first |
106.7252288 |
0.4131318 |
NA |
0.3717260 |
cas |
[0, 5) |
secon |
102.6018363 |
0.6523066 |
-0.0020711 |
0.9608153 |
cas |
[5, 40) |
null |
125.3399596 |
NA |
NA |
NA |
cas |
[5, 40) |
first |
123.1233110 |
0.1166657 |
NA |
0.3657985 |
cas |
[5, 40) |
secon |
105.0956672 |
0.4527831 |
-0.0010058 |
0.0812513 |
cas |
[40, 80) |
null |
125.3399596 |
NA |
NA |
NA |
cas |
[40, 80) |
first |
123.9213204 |
0.0992055 |
NA |
0.7843676 |
cas |
[40, 80) |
secon |
101.3922279 |
0.5292657 |
-0.0010270 |
0.0736023 |
ctc |
[0, 5) |
null |
125.3399596 |
NA |
NA |
NA |
ctc |
[0, 5) |
first |
18.1834217 |
9.3431233 |
NA |
0.0000000 |
ctc |
[0, 5) |
secon |
-35.5895227 |
21.4547573 |
-0.5987012 |
0.1064256 |
ctc |
[5, 40) |
null |
125.3399596 |
NA |
NA |
NA |
ctc |
[5, 40) |
first |
77.3971759 |
6.9469844 |
NA |
0.0755217 |
ctc |
[5, 40) |
secon |
19.3268654 |
21.8761331 |
-0.7893185 |
0.1702872 |
ctc |
[40, 80) |
null |
125.3399596 |
NA |
NA |
NA |
ctc |
[40, 80) |
first |
33.6261271 |
18.1252633 |
NA |
0.0000055 |
ctc |
[40, 80) |
secon |
-24.9270705 |
43.2765769 |
-2.6088990 |
0.4969509 |
I |
[0, 5) |
null |
130.7281877 |
NA |
NA |
NA |
I |
[0, 5) |
first |
142.5518578 |
-19.0085912 |
NA |
0.0346537 |
I |
[0, 5) |
secon |
143.5441724 |
18.8677215 |
-43.0403917 |
0.9555452 |
I |
[5, 40) |
null |
130.7281877 |
NA |
NA |
NA |
I |
[5, 40) |
first |
136.9520601 |
-12.6513906 |
NA |
0.8787615 |
I |
[5, 40) |
secon |
118.5823132 |
44.7030139 |
-40.6825566 |
0.9852954 |
I |
[40, 80) |
null |
125.3399596 |
NA |
NA |
NA |
I |
[40, 80) |
first |
136.8050670 |
-10.2689290 |
NA |
0.5068023 |
I |
[40, 80) |
secon |
122.3674928 |
27.0594748 |
-21.8517283 |
0.8107026 |
k |
[0, 5) |
null |
125.3399596 |
NA |
NA |
NA |
k |
[0, 5) |
first |
94.8403859 |
0.2905706 |
NA |
0.3161960 |
k |
[0, 5) |
secon |
64.7081240 |
0.9089478 |
-0.0024286 |
0.5565028 |
k |
[5, 40) |
null |
125.3399596 |
NA |
NA |
NA |
k |
[5, 40) |
first |
98.6329934 |
1.0351537 |
NA |
0.1853189 |
k |
[5, 40) |
secon |
78.3275639 |
2.4080313 |
-0.0193771 |
0.2078543 |
k |
[40, 80) |
null |
125.3399596 |
NA |
NA |
NA |
k |
[40, 80) |
first |
114.2123355 |
0.2483351 |
NA |
0.2088884 |
k |
[40, 80) |
secon |
110.2704307 |
0.5279234 |
-0.0017614 |
0.9900008 |
mg |
[0, 5) |
null |
125.3399596 |
NA |
NA |
NA |
mg |
[0, 5) |
first |
88.3719963 |
24.1620676 |
NA |
0.0056628 |
mg |
[0, 5) |
secon |
63.7110559 |
47.5122765 |
-4.7267629 |
0.3424041 |
mg |
[5, 40) |
null |
125.3399596 |
NA |
NA |
NA |
mg |
[5, 40) |
first |
98.3412154 |
20.6097284 |
NA |
0.0000000 |
mg |
[5, 40) |
secon |
90.7965741 |
30.5876948 |
-2.6183831 |
0.4430848 |
mg |
[40, 80) |
null |
125.3399596 |
NA |
NA |
NA |
mg |
[40, 80) |
first |
97.4437881 |
35.1025581 |
NA |
0.0094896 |
mg |
[40, 80) |
secon |
93.0788384 |
62.5756826 |
-16.4722335 |
0.0127413 |
mo |
[0, 5) |
null |
125.3399596 |
NA |
NA |
NA |
mo |
[0, 5) |
first |
80.6395689 |
0.9628748 |
NA |
0.0000026 |
mo |
[0, 5) |
secon |
92.4213577 |
0.4386504 |
0.0042559 |
0.4313433 |
mo |
[5, 40) |
null |
125.3399596 |
NA |
NA |
NA |
mo |
[5, 40) |
first |
90.4940904 |
2.1278751 |
NA |
0.0028011 |
mo |
[5, 40) |
secon |
90.4940904 |
2.3889639 |
-0.0099522 |
0.7652067 |
mo |
[40, 80) |
null |
125.3399596 |
NA |
NA |
NA |
mo |
[40, 80) |
first |
54.5965946 |
7.4466700 |
NA |
0.0002254 |
mo |
[40, 80) |
secon |
-17.6631983 |
22.2022824 |
-0.6489471 |
0.0135526 |
n |
[0, 5) |
null |
130.7281877 |
NA |
NA |
NA |
n |
[0, 5) |
first |
106.1275835 |
7.5803934 |
NA |
0.5878307 |
n |
[0, 5) |
secon |
343.5861245 |
-141.0437397 |
21.6700783 |
0.0657778 |
n |
[5, 40) |
null |
130.7281877 |
NA |
NA |
NA |
n |
[5, 40) |
first |
56.6455414 |
30.3521323 |
NA |
0.0968684 |
n |
[5, 40) |
secon |
-3.1554711 |
93.4554648 |
-14.8056498 |
0.1981882 |
n |
[40, 80) |
null |
125.3399596 |
NA |
NA |
NA |
n |
[40, 80) |
first |
150.1322667 |
-11.2830135 |
NA |
0.4630350 |
n |
[40, 80) |
secon |
147.2798058 |
-8.6197431 |
-0.5841696 |
0.9905613 |
p |
[0, 5) |
null |
130.7281877 |
NA |
NA |
NA |
p |
[0, 5) |
first |
110.8585205 |
1.7298968 |
NA |
0.0498837 |
p |
[0, 5) |
secon |
104.1407964 |
5.5962157 |
-0.1586961 |
0.7864525 |
p |
[5, 40) |
null |
125.3399596 |
NA |
NA |
NA |
p |
[5, 40) |
first |
120.6124191 |
2.8479160 |
NA |
0.5378798 |
p |
[5, 40) |
secon |
132.7182502 |
-10.1819150 |
1.1580122 |
0.4553431 |
p |
[40, 80) |
null |
125.3399596 |
NA |
NA |
NA |
p |
[40, 80) |
first |
123.5466402 |
4.3739498 |
NA |
0.3679250 |
p |
[40, 80) |
secon |
131.0294212 |
-30.1789505 |
5.5598812 |
0.6625321 |
ph |
[0, 5) |
null |
125.3399596 |
NA |
NA |
NA |
ph |
[0, 5) |
first |
-185.2818359 |
45.4089038 |
NA |
0.0038973 |
ph |
[0, 5) |
secon |
-228.3331060 |
58.1992239 |
-0.9474311 |
0.9650765 |
ph |
[5, 40) |
null |
125.3399596 |
NA |
NA |
NA |
ph |
[5, 40) |
first |
-78.1712241 |
32.5016237 |
NA |
0.0097559 |
ph |
[5, 40) |
secon |
-419.2027386 |
146.7780169 |
-9.4301029 |
0.4019841 |
ph |
[40, 80) |
null |
125.3399596 |
NA |
NA |
NA |
ph |
[40, 80) |
first |
-3.0427377 |
21.1423992 |
NA |
0.2350978 |
ph |
[40, 80) |
secon |
216.9607180 |
-54.7428507 |
6.3769118 |
0.7749197 |
S |
[0, 5) |
null |
130.7281877 |
NA |
NA |
NA |
S |
[0, 5) |
first |
97.1033864 |
-137.1148424 |
NA |
0.1934667 |
S |
[0, 5) |
secon |
230.1793191 |
727.4623888 |
1148.2501243 |
0.0526720 |
S |
[5, 40) |
null |
130.7281877 |
NA |
NA |
NA |
S |
[5, 40) |
first |
112.8938051 |
-115.4770425 |
NA |
0.4502783 |
S |
[5, 40) |
secon |
95.5808004 |
-358.6291512 |
-765.6294102 |
0.7286439 |
S |
[40, 80) |
null |
125.3399596 |
NA |
NA |
NA |
S |
[40, 80) |
first |
135.8980053 |
54.9045455 |
NA |
0.6150499 |
S |
[40, 80) |
secon |
134.0359725 |
29.3864320 |
-60.9911750 |
0.9831038 |
sat |
[0, 5) |
null |
125.3399596 |
NA |
NA |
NA |
sat |
[0, 5) |
first |
-19.7848062 |
1.8621851 |
NA |
0.0000014 |
sat |
[0, 5) |
secon |
-19.2717225 |
1.8346242 |
0.0002483 |
0.9962725 |
sat |
[5, 40) |
null |
125.3399596 |
NA |
NA |
NA |
sat |
[5, 40) |
first |
30.7137363 |
1.4601676 |
NA |
0.0021402 |
sat |
[5, 40) |
secon |
40.8642741 |
0.9048139 |
0.0052851 |
0.8377722 |
sat |
[40, 80) |
null |
125.3399596 |
NA |
NA |
NA |
sat |
[40, 80) |
first |
83.7054805 |
0.8394767 |
NA |
0.1031402 |
sat |
[40, 80) |
secon |
106.9974701 |
-0.5201477 |
0.0145440 |
0.6106124 |
Ui |
[0, 5) |
null |
130.7281877 |
NA |
NA |
NA |
Ui |
[0, 5) |
first |
74.0904730 |
125.8978534 |
NA |
0.6683206 |
Ui |
[0, 5) |
secon |
-0.3864461 |
510.0777980 |
-470.2272890 |
0.7771003 |
Ui |
[5, 40) |
null |
130.7281877 |
NA |
NA |
NA |
Ui |
[5, 40) |
first |
189.9649650 |
-120.1787644 |
NA |
0.5702511 |
Ui |
[5, 40) |
secon |
-214.8193626 |
1553.1463106 |
-1711.9311693 |
0.8092556 |
Ui |
[40, 80) |
null |
125.3399596 |
NA |
NA |
NA |
Ui |
[40, 80) |
first |
90.5095782 |
61.5251262 |
NA |
0.7255424 |
Ui |
[40, 80) |
secon |
-263.1545532 |
1486.3876744 |
-1402.1356242 |
0.3999665 |
Ur |
[0, 5) |
null |
130.7281877 |
NA |
NA |
NA |
Ur |
[0, 5) |
first |
146.7536272 |
-95.2672771 |
NA |
0.5950321 |
Ur |
[0, 5) |
secon |
70.5667213 |
660.7814220 |
-1775.0689420 |
0.2109792 |
Ur |
[5, 40) |
null |
130.7281877 |
NA |
NA |
NA |
Ur |
[5, 40) |
first |
96.4979559 |
165.7179692 |
NA |
0.2342710 |
Ur |
[5, 40) |
secon |
91.9034604 |
311.3371599 |
-597.2970319 |
0.8605253 |
Ur |
[40, 80) |
null |
125.3399596 |
NA |
NA |
NA |
Ur |
[40, 80) |
first |
100.5071318 |
86.3463986 |
NA |
0.5660589 |
Ur |
[40, 80) |
secon |
43.5272675 |
615.9799131 |
-1048.1004065 |
0.5375682 |
Us |
[0, 5) |
null |
130.7281877 |
NA |
NA |
NA |
Us |
[0, 5) |
first |
36.5036707 |
147.4547824 |
NA |
0.1931430 |
Us |
[0, 5) |
secon |
-366.7180069 |
1545.6595871 |
-1200.3176207 |
0.7455504 |
Us |
[5, 40) |
null |
130.7281877 |
NA |
NA |
NA |
Us |
[5, 40) |
first |
261.8051853 |
-200.3486456 |
NA |
0.1899799 |
Us |
[5, 40) |
secon |
-598.5709640 |
2242.0511630 |
-1719.6932484 |
0.8029661 |
Us |
[40, 80) |
null |
125.3399596 |
NA |
NA |
NA |
Us |
[40, 80) |
first |
222.9996526 |
-139.9538365 |
NA |
0.4909162 |
Us |
[40, 80) |
secon |
-191.6242472 |
883.2021409 |
-614.2777354 |
0.7484711 |
al |
[5, 40) |
first |
137.12138 |
-105.5689730 |
NA |
0.0018894 |
al |
[40, 80) |
first |
137.12138 |
-66.2268338 |
NA |
0.0292740 |
alp |
[5, 40) |
first |
120.38098 |
-28.6306365 |
NA |
0.0015285 |
ca |
[0, 5) |
secon |
28.27715 |
24.0039433 |
-1.0808063 |
0.0338630 |
ca |
[5, 40) |
secon |
36.84704 |
43.1311267 |
-3.4654478 |
0.0219065 |
ctc |
[0, 5) |
first |
18.18342 |
9.3431233 |
NA |
0.0000000 |
ctc |
[40, 80) |
first |
33.62613 |
18.1252633 |
NA |
0.0000055 |
I |
[0, 5) |
first |
142.55186 |
-19.0085912 |
NA |
0.0346537 |
mg |
[0, 5) |
first |
88.37200 |
24.1620676 |
NA |
0.0056628 |
mg |
[5, 40) |
first |
98.34122 |
20.6097284 |
NA |
0.0000000 |
mg |
[40, 80) |
secon |
93.07884 |
62.5756826 |
-16.4722335 |
0.0127413 |
mo |
[0, 5) |
first |
80.63957 |
0.9628748 |
NA |
0.0000026 |
mo |
[5, 40) |
first |
90.49409 |
2.1278751 |
NA |
0.0028011 |
mo |
[40, 80) |
secon |
-17.66320 |
22.2022824 |
-0.6489471 |
0.0135526 |
p |
[0, 5) |
first |
110.85852 |
1.7298968 |
NA |
0.0498837 |
ph |
[0, 5) |
first |
-185.28184 |
45.4089038 |
NA |
0.0038973 |
ph |
[5, 40) |
first |
-78.17122 |
32.5016237 |
NA |
0.0097559 |
sat |
[0, 5) |
first |
-19.78481 |
1.8621851 |
NA |
0.0000014 |
sat |
[5, 40) |
first |
30.71374 |
1.4601676 |
NA |
0.0021402 |
# Limites de recomendação.
# Torância: 10% abaixo da maior produção observada.
tol <- max(teca_arv$prod) - max(teca_arv$prod) * 0.9
quantile_limits <- function(beta_0, beta_1, beta_2, tol, x_range) {
coefs <- c(c = beta_0, b = beta_1, a = beta_2)
# ex <- extendrange(x_range)
ex <- x_range
x_seq <- seq(ex[1], ex[2], length.out = 51)
if (is.finite(beta_2)) {
# Modelo de segundo grau.
x_opt <- -beta_1/(2 * beta_2)
y_opt <- c(x_opt^(0:2) %*% coefs)
roots <- with(as.list(coefs), {
delta <- sqrt(b^2 - 4 * a * (c - y_opt + tol))
suppressWarnings({
(-b + c(-1, 1) * delta)/(2 * a)
})
})
limits <- data.frame(y_opt = y_opt,
x_opt = x_opt,
y_tol = y_opt - tol,
x_tol_inf = min(roots),
x_tol_sup = max(roots))
pred <- data.frame(x = x_seq,
y = beta_0 + beta_1 * x_seq + beta_2 * x_seq^2)
return(list(limits, pred))
} else if (is.finite(beta_1)) {
# Modelo de primeiro grau.
y_range <- beta_0 + beta_1 * x_range
x_opt <- x_range[which.max(y_range)]
y_opt <- max(y_range)
x_tol <- x_opt - tol/beta_1
limits <- data.frame(y_opt = y_opt,
x_opt = x_opt,
y_tol = y_opt - tol,
x_tol_inf = ifelse(x_tol < x_opt, x_tol, NA),
x_tol_sup = ifelse(x_tol > x_opt, x_tol, NA))
pred <- data.frame(x = x_seq,
y = beta_0 + beta_1 * x_seq)
return(list(limits, pred))
} else {
# Modelo nulo.
pred <- data.frame(x = x_seq,
y = beta_0)
limits <- data.frame(y_opt = beta_0,
x_opt = NA,
y_tol = beta_0 - tol,
x_tol_inf = NA,
x_tol_sup = NA)
return(list(limits, pred))
}
}
# Amplitude das variáveis preditoras.
tb_range <- tb_long %>%
drop_na() %>%
group_by(variable, cam) %>%
summarise(x_range = list(range(valor)))
# O modelo para cada camada com prioridade secon > first > null.
tb_model <- tb_result %>%
filter(pvalue < 0.05 | is.na(pvalue)) %>%
group_by(variable, cam) %>%
slice(n()) %>%
ungroup()
# xtabs(~variable + cam, data = tb_model)
# Junta com a informação de amplitude das variáveis.
tb_limits <- inner_join(tb_model, tb_range)
## Joining, by = c("variable", "cam")
# Obtém os limites e a tabela de valores preditos.
tb_limits <- tb_limits %>%
mutate(limits = pmap(list(beta_0,
beta_1,
beta_2,
tol = tol,
x_range = x_range),
quantile_limits))
tb_limits
## # A tibble: 63 x 9
## variable cam model beta_0 beta_1 beta_2 pvalue x_range limits
## <chr> <fct> <fct> <dbl> <dbl> <dbl> <dbl> <list> <list>
## 1 acc [0, … null 125. NA NA NA <dbl [… <list…
## 2 acc [5, … null 125. NA NA NA <dbl [… <list…
## 3 acc [40,… null 125. NA NA NA <dbl [… <list…
## 4 al [0, … null 125. NA NA NA <dbl [… <list…
## 5 al [5, … first 137. -106. NA 0.00189 <dbl [… <list…
## 6 al [40,… first 137. -66.2 NA 0.0293 <dbl [… <list…
## 7 alp [0, … null 131. NA NA NA <dbl [… <list…
## 8 alp [5, … first 120. -28.6 NA 0.00153 <dbl [… <list…
## 9 alp [40,… null 125. NA NA NA <dbl [… <list…
## 10 are [0, … null 125. NA NA NA <dbl [… <list…
## # … with 53 more rows
# Variávies para traçar as linhas de orientação.
tb_lines <- tb_limits %>%
select(variable, cam, limits) %>%
mutate(limits = map(limits, `[[`, 1)) %>%
unnest(limits)
# xtabs(~variable + cam, data = tb_lines)
# Valores preditos de acordo com os modelos escolhidos.
tb_pred <- tb_limits %>%
select(variable, cam, limits) %>%
mutate(limits = map(limits, `[[`, 2)) %>%
unnest(limits)
# xtabs(~variable + cam, data = tb_pred)
# Gráfico.
ggplot(data = tb_long,
mapping = aes(x = valor, y = prod, color = cam)) +
facet_wrap(facets = ~variable, scales = "free_x", ncol = 3) +
geom_point(shape = 1) +
# ylim(extendrange(teca_arv$prod)) +
labs(color = "Camada") +
xlab("Valor da variável de solo") +
ylab("Produção de teca") +
# geom_quantile(quantiles = 0.9,
# formula = y ~ poly(x, degree = 2),
# linetype = 2) +
geom_line(data = tb_pred,
mapping = aes(x = x, y = y, color = cam),
size = 1.25) +
# geom_hline(data = tb_lines,
# mapping = aes(yintercept = y_opt, color = cam),
# linetype = 2) +
# geom_hline(data = tb_lines,
# mapping = aes(yintercept = y_tol, color = cam),
# linetype = 3) +
geom_vline(data = tb_lines,
mapping = aes(xintercept = x_opt, color = cam)) +
geom_vline(data = tb_lines,
mapping = aes(xintercept = x_tol_inf, color = cam),
linetype = 4) +
geom_vline(data = tb_lines,
mapping = aes(xintercept = x_tol_sup, color = cam),
linetype = 4) +
theme()
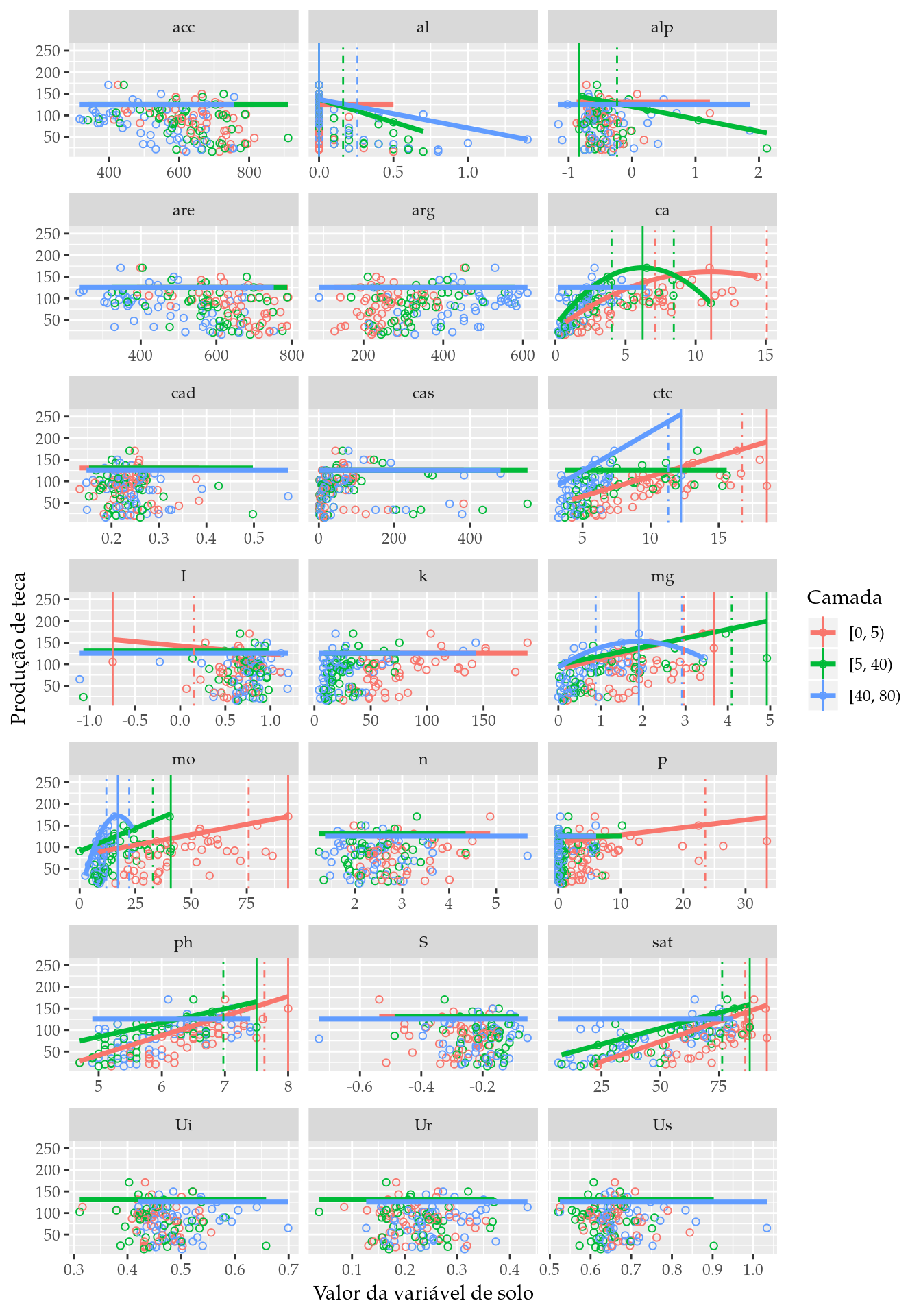